Explore the power of AI to predict trends, optimize strategies, and gain a competitive edge. Discover the leading Predictive AI tools shaping the future of business.
Let’s be honest, wouldn’t it be awesome to have a little heads-up about the future? Well, as someone who’s dabbled in everything from marketing analytics to the occasional stock market foray, I’ve always been fascinated by the idea of prediction.
That’s why predictive AI tools feel like my own personal crystal ball. They’re changing the game across industries, and I’m here to spill the beans on my top 10 favorites.
Why Predictive AI?
Predictive AI isn’t magic. It’s the power of machine learning applied to historical data to uncover patterns, trends, and probabilities.
Imagine feeding a predictive model all your past customer interactions – it starts figuring out who’s likely to buy, who might need a little nudge, and even who’s about to jump ship.
This superpower lets businesses make smarter decisions, saving time and money while turning “maybes” into “definitelys.”
What is Predictive AI?
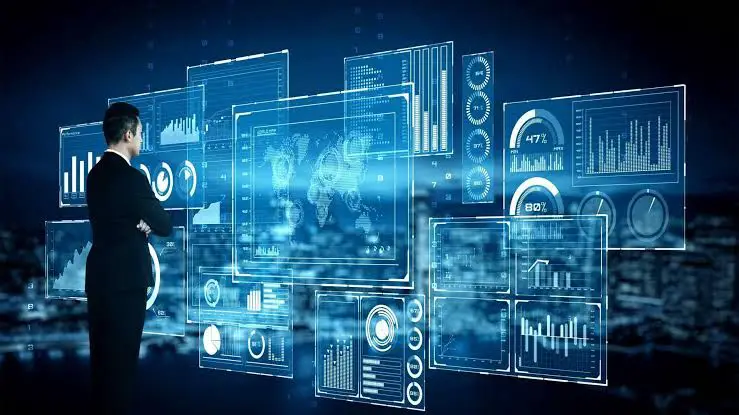
Predictive AI is a branch of artificial intelligence that leverages machine learning algorithms and statistical techniques to analyze historical data and identify patterns. These patterns are then used to make informed predictions about future events or behaviors.
How does Predictive AI work?
- Data Collection and Preparation: Predictive AI begins with gathering relevant data from various sources (sales records, customer interactions, sensor readings, etc.). This data is cleaned and organized to ensure quality predictive analysis.
- Model Development: Machine learning algorithms are selected and trained on this historical data. These algorithms learn to identify patterns and correlations that exist within the dataset.
- Prediction: Once the model is trained, it can be fed new data to generate predictions. These predictions can range from customer churn likelihood to equipment failure probabilities to sales forecasts.
Key Applications of Predictive AI:
- Risk Assessment: Identify potential risks like customer churn, fraudulent transactions, or equipment malfunctions, enabling proactive mitigation.
- Demand Forecasting: Anticipate future demand for products or services, optimizing inventory management and resource allocation.
- Customer Behavior Analysis: Understand customer preferences and purchasing patterns, enabling personalized marketing and improved customer experience.
- Predictive Maintenance: Predict potential failures in machinery or infrastructure, allowing for proactive maintenance and preventing costly downtime.
Benefits of Predictive AI
- Data-driven decision making: Provides insights to support strategic choices and optimize business operations.
- Improved efficiency: Automates predictions and pattern discovery, saving time and resources.
- Enhanced risk management: Reduces potential losses by allowing proactive intervention.
- Competitive Advantage: Companies gain an edge by anticipating and responding to emerging trends before their competitors.
Top 10 Predictive AI Tools
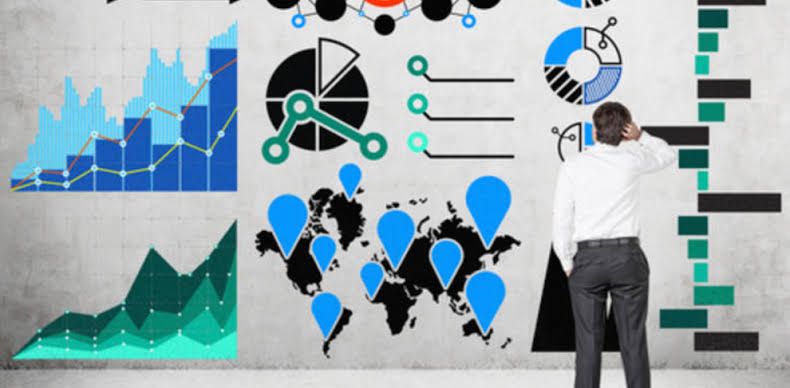
1. RapidMiner
My first serious predictive AI fling was with RapidMiner. Remember that time I wanted to predict which email campaigns would be most successful?
We had a mountain of data – open rates, click-throughs, time of day, you name it. RapidMiner let me drag, drop, and connect different analysis modules.
Sure, I fumbled through it at first, but by the end, I had a model that actually helped us send the right emails at the right time. Result: way better engagement!
2. IBM SPSS
Now, SPSS is the granddaddy of statistical analysis, dressed up with predictive AI capabilities. I’ve only dipped my toes in, but even a newbie like me could sense the power.
If you have massive datasets and a team that knows their statistics, SPSS is your beast. (Just a heads-up, it ain’t the most beginner-friendly).
3. Alteryx
Picture Alteryx as a super-powered data prep machine that moonlights as a predictive modeling tool. Back when I was wrangling messy, chaotic customer data, Alteryx was a lifesaver.
It cleans, blends, and gets everything sparkly and organized for you. Plus, it has predictive features built-in. It saved me from a spreadsheet nightmare!
4. Google Cloud BigQuery
BigQuery is my go-to if I’m dealing with massive datasets (and honestly, who isn’t these days?).
It’s part of Google’s cloud services, making it super scalable. Now, it’s not drag-and-drop simple, you’ll need some SQL skills. But if you handle a ton of information and need insights fast, BigQuery is a winner.
5. Microsoft Azure Machine Learning
My company is team Microsoft for life, so I’d be remiss not to mention their Azure Machine Learning.
It’s cloud-based, which means easy collaboration, and if you’re familiar with other Azure tools, it fits right in with your existing setup.
I particularly like their focus on simplifying the deployment of predictive models into actual business applications, which turns all that data magic into actionable results.
6. Dataiku
If you want the whole shebang, Dataiku is your AI orchestra. From data prep to model building to deployment, it’s an end-to-end platform.
It’s seriously impressive, but remember, with great power comes complexity. Dataiku is best if you have a data science team that can truly harness its capabilities.
7. SAP Predictive Analytics
Let’s say you’re a big enterprise running SAP systems – their Predictive Analytics offering is a logical choice. It integrates seamlessly, and if you’re focused on business-centric insights (think: forecasting sales, predicting equipment failures), it’s worth exploring. My only caveat – it gets pretty pricey!
8. Qlik Sense
Qlik Sense is known for gorgeous data visualizations. Add their predictive superpowers, and bam – dashboards that practically give you the future.
I once used it to analyze sales trends with a dash of customer sentiment sprinkled in. The results were stunning. I could almost see the opportunities popping out.
9. Sisense
Another platform strong in visualization, Sisense makes predictive insights feel almost effortless. It’s especially good for handling complex data from different sources. We recently used it to combine website activity, social media buzz, and sales figures for a clearer picture of our campaign performance. The ‘what-if’ analysis features really blew my mind!
10. KNIME
Open-source for the win! If you’re budget-conscious or like to tinker under the hood, KNIME is a fantastic choice. It might seem intimidating at first, but the community is huge, so there’s tons of support. While I was experimenting with churn prediction for a subscription service, KNIME’s flexibility let me try out all sorts of different algorithms. True DIY data science!
Pricing/Cost Considerations of Predictive AI
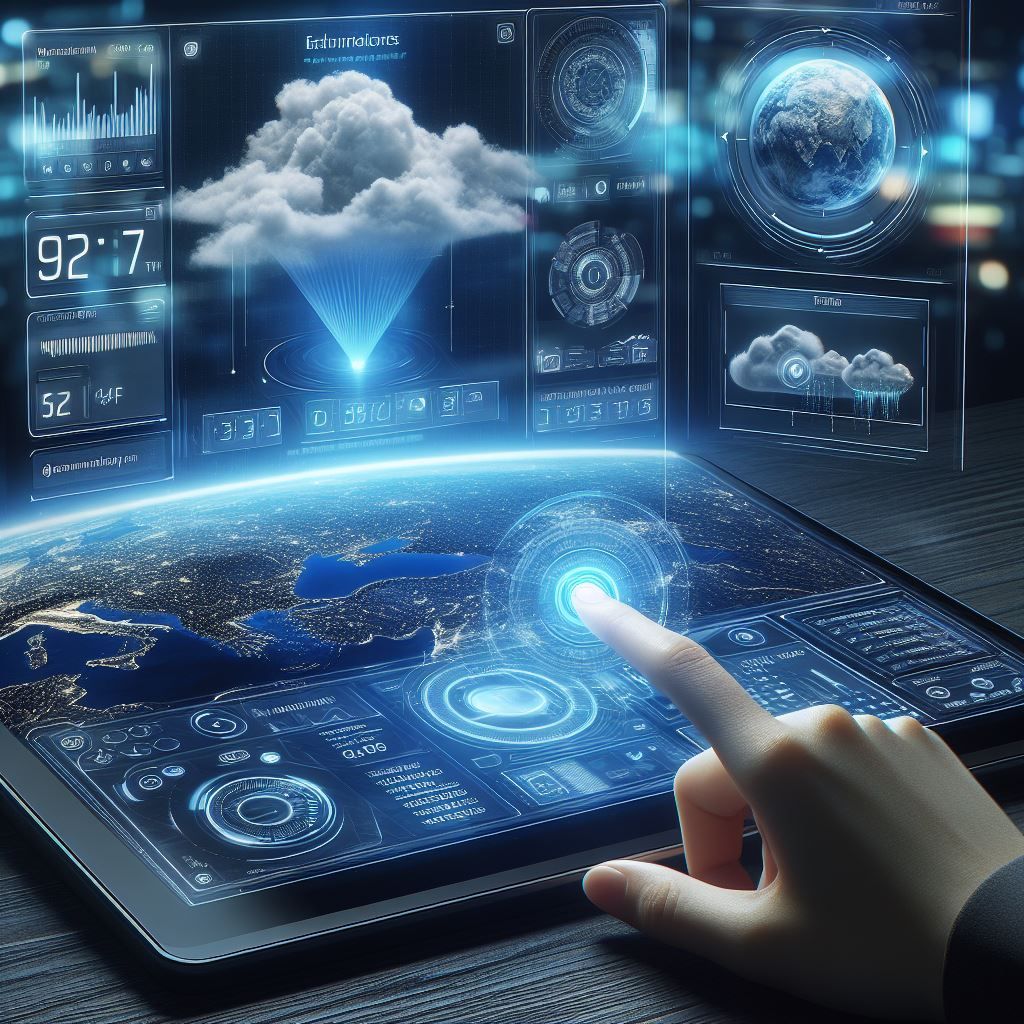
Predictive AI tools come with a range of price tags. Here’s a quick breakdown of the factors to keep in mind:
- Open-source vs. Paid: Open-source tools like KNIME are free to use, but may require more technical expertise to set up and manage. Paid platforms offer user-friendliness and support, but with monthly or annual fees.
- Cloud vs. On-premise: Cloud-based solutions (BigQuery, Azure Machine Learning) are scalable and pay-as-you-go. On-premise software can give you more control, but you’ll have upfront and maintenance costs for your own infrastructure.
- Model Complexity: Larger, more complex datasets and elaborate models tend to drive up costs, especially for cloud-based services.
Use Case Examples of Predictive AI Tools
Let’s see how Predictive AI gets down to business:
- Retailer: Inventory Optimization A predictive model analyzes sales trends, seasonality, even weather data to predict demand. Result: Stockouts are avoided, overstocking is reduced, and profits improve.
- Manufacturer: Predictive Maintenance Sensors on machinery feed into a model that predicts the likelihood of breakdowns. This allows maintenance to be scheduled proactively, preventing costly downtime.
- Healthcare: Personalized Treatment Plans Analyzing patient data, medical histories, and research trends, a predictive tool helps doctors suggest treatments with a higher probability of success, improving patient outcomes.
The Truth About Predictive AI
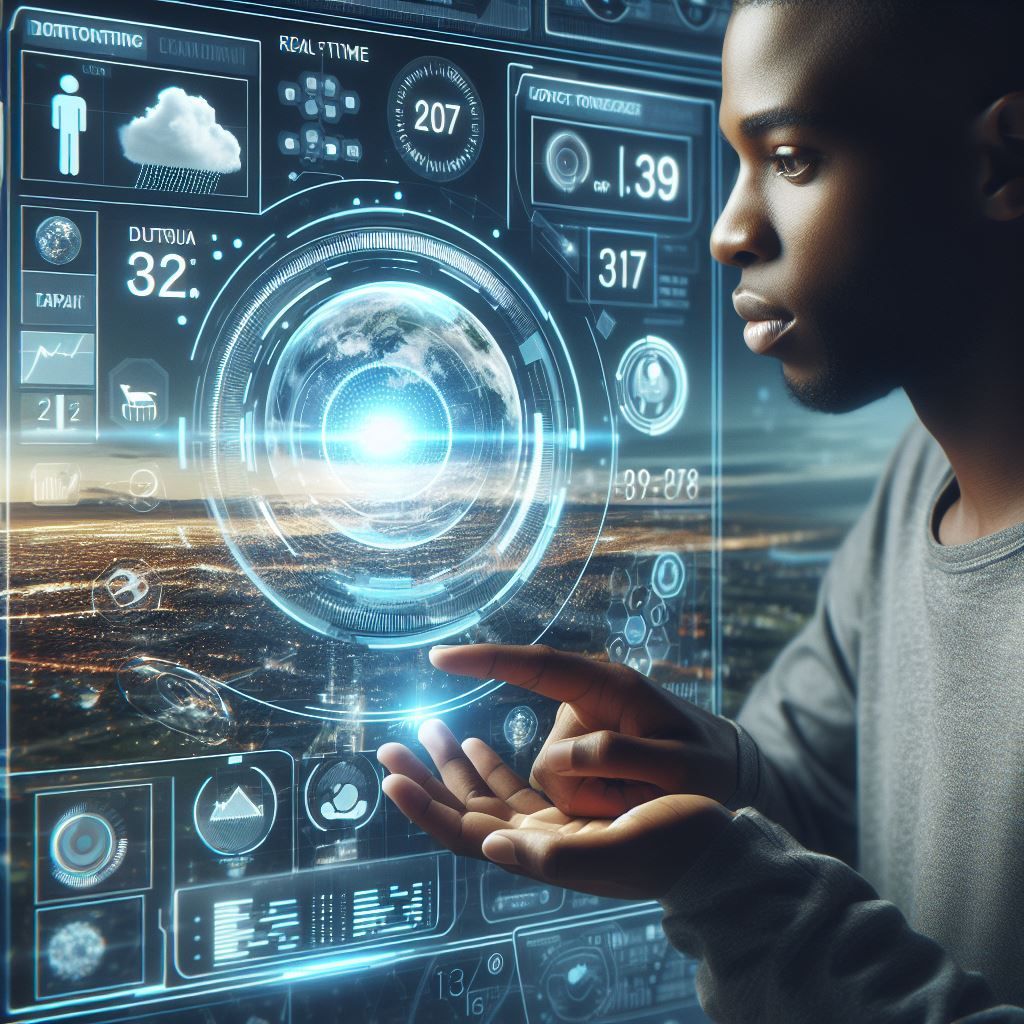
Here’s the thing about predictive AI:
- No garbage in, no gold out: Your models are only as good as the data they’re trained on. Invest in clean, accurate data – it’ll make your life so much easier.
- Predictions aren’t guarantees: Predictive AI deals with probabilities, not certainties. It’s a guide, not a dictator. It’s how you use the insights that brings home the bacon.
- Start small, scale up: Don’t try to build a doomsday-prediction machine right out of the gate. Pick a focused business problem, experiment, and then expand.
A Personal Prediction…
I’m not just excited about these tools because I’m a geek. Seriously, I believe predictive AI has the power to transform the way we do business across every industry. Here’s how I see it:
- Smarter decisions, less guesswork: Imagine having an AI advisor to weigh in on everything from marketing campaigns to investment opportunities. It’s like having an extra brain in the room.
- Hyper-personalization at scale: Knowing your customers’ likely next move lets you tailor experiences. We ain’t talking cheesy, generic recommendations – it’s about offering what people really want, before they even ask.
- Efficiency boost to the max: From predicting equipment failures before they happen to streamlining complex processes, AI saves time, money, and a whole lot of headaches.
The Future is Predictably Bright
Predictive AI isn’t science fiction anymore. It’s real, it’s accessible, and it’s changing business (and maybe even my attempts to play the stock market!). The tools on this list are just the beginning. As AI advances, I can’t wait to see how “predicting the future” will become as common as checking the weather.
So there you have it – my top 10 predictive AI tools and a glimpse into why I’m convinced this is a tech revolution worth paying attention to. Have you tried any of these tools yet? Let me know your favorites in the comments!
Let me know if you’d like any additions or changes made!
Predictive AI FAQs
Q: What exactly is predictive AI?
A: Think of predictive AI as a crystal ball powered by statistics and machine learning. It analyzes massive amounts of historical data to find patterns, uncover trends, and ultimately forecast what’s likely to happen in the future. Businesses use this to get an edge, whether it’s predicting sales, customer behavior, or potential equipment breakdowns.
Q: How does predictive AI actually work?
A: Here’s a simplified breakdown:
- Data Feast: Predictive AI models gobble up vast amounts of data – your sales records, website traffic, weather patterns, you name it. The more data and the better the quality, the smarter the predictions.
- Pattern Hunters: The AI uses statistical techniques and machine learning algorithms to search for relationships and patterns in the data. It’s like meticulously studying a giant puzzle for clues.
- Behold, the Future! (Kinda): Based on those patterns, the model predicts what is likely to happen. That could be the probability of a customer churning, the risk of a machine part failing, or the expected demand for a new product.
Q: Okay, but what are some real-world examples of predictive AI in action?
A: It’s everywhere! Here are just a few:
- Retail: Predicting which products will fly off the shelves, optimizing inventory, personalizing product recommendations.
- Finance: Detecting fraudulent transactions, assessing loan risk, predicting market fluctuations.
- Healthcare: Predicting the likelihood of diseases, improving diagnoses, personalizing treatment plans.
- Manufacturing: Forecasting equipment failure (preventing costly downtime), optimizing production schedules.
- Customer service: Predicting when customers might need help, routing inquiries to the right agent for quicker resolution.
Q: This sounds cool, but is it accurate?
A: Predictive AI is never 100% accurate. It’s about probabilities and informed guesses. However, with good data and the right models, predictive AI consistently outperforms traditional guesswork. It’s about making smarter, data-driven decisions rather than relying on gut feelings.
Q: Can any business just jump into using predictive AI?
A: Not quite. Here’s what you need:
- Data, data, data: Without a rich historical dataset, there’s nothing for the models to analyze.
- Expertise: Setting up and refining predictive models often requires data scientists or specialized AI platforms.
- A Focused Goal: You need to know what you want to predict and how you’ll use those predictions to make better decisions.
Q: What are the ethical concerns surrounding predictive AI?
A: Like any powerful technology, predictive AI needs guardrails:
- Bias: If the data used to train models is biased, the predictions will be too, potentially causing harm.
- Privacy: Predictive AI needs lots of data, raising questions about how that data is collected and used.
- Transparency: Sometimes these models can be a ‘black box’, leading to a lack of trust in their outputs. Ensuring explanations behind the predictions is key.
Discover more from Digital Wealth Guru
Subscribe to get the latest posts sent to your email.
Comments